Can behaviour-monitoring devices predict calving or metabolic disease?
- Guest Writer
- Aug 5, 2020
- 7 min read
Milk fever and ketosis are common metabolic diseases during the transition period (the 6‑week period surrounding calving).
Stacey Hendriks has just completed her PhD in Animal Science at Massey University focused on identifying subclinical disease in dairy cows using wearable behaviour-monitoring technologies with the goal of supporting farmers to prevent illness, and improve health, welfare, and productivity. We have asked Stacey to provide a summary of some of her research using these behaviour-monitoring devices.
Key Points
Wearable behaviour-monitoring devices are already on the market and have potential to predict onset of calving and metabolic disease, as well as cows on heat.
Heat detection using wearable devices is well supported by scientific studies.
Predicting when a dairy cow will calve or become sick has potential, but further research is needed.
Milk fever usually occurs at calving when a dairy cow is unable to adapt to the sudden, increased demand for calcium to support milk production. Clinical milk fever (e.g. a downer cow) occurs in approximately 3-5% of grazing dairy cows; however, subclinical hypocalcaemia, characterised by low blood calcium concentrations, affects, on average, 30 to 50% of the herd (Roche, 2003; Roberts and McDougall, 2019).
Ketosis occurs during severe negative energy balance when a dairy cow’s liver is unable to fully process the large quantity of circulating mobilised body fat, and produces ketones, namely β-hydroxybutyrate, as an alternative energy source to support milk production. While clinical ketosis is relatively uncommon, subclinical ketosis can affect 68 to 75% of cows in the first 5 weeks post-calving (Phyn et al., 2017).
The high prevalence of these subclinical diseases in grazing dairy cows and potential impacts on cow performance and health, support a focus on developing new tools to improve the identification of cows at risk of metabolic disease.
Monitoring disease
Animals display common sickness behaviours such as reduced appetite, depression, lethargy, and discomfort, which farmers observe to identify when an animal is unwell and experiencing a clinical illness. More recently, scientists have identified that subtle changes in lying behaviour and activity that are difficult to observe, but can be measured remotely using behaviour‑monitoring devices, could assist in the early identification of disease.
Figure 1. A leg-worn (a), neck-worn (b) (Source: DairyNZ), and an ear tag monitor (c) (Source: https://herddogg.com/).
Since interventions are typically more successful if they start earlier in the disease process, e.g. before clinical signs, these technologies may allow for improved treatment and management of at-risk and sick animals.
Several wearable devices are already on the market including neck‑worn collars, leg bands, and ear tags that can record a range of lying behaviours and activity (see Figure 1 above). These technologies are particularly relevant for large herds where time to observe individual animals is limited, and in situations where farm staff are inexperienced in animal husbandry.
Our research funded by DairyNZ and MBIE was the first to use leg-attached devices (Figure 1a) to characterise the lying behaviour and activity of grazing dairy cows experiencing subclinical forms of milk fever and ketosis.
Ketosis
In our study, cows with subclinical ketosis (high blood ketone and fatty acid levels) during the first 2 weeks post-calving (Figure 2):
spent less time lying;
walked more; and,
transitioned less frequently between lying and standing, compared with healthy cows in the 3 weeks before diagnosis (~ 5 days post-calving).
The graph on the left shows the average time cows spent lying per day in the 3-weeks leading up to calving and the 3-weeks after calving for cows that had subclinical ketosis (purple) or were healthy (black). The graph on the right shows the average number of steps cows took per day before and after calving.
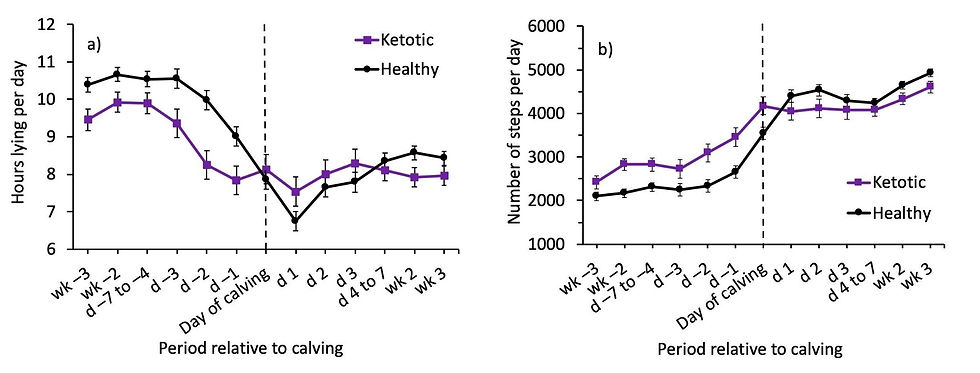
Milk fever (hypocalcaemia)
In a separate study, we found that cows with severe hypocalcaemia (very low blood calcium levels; ≤1.4 mmol/L without clinical milk fever within 48 hours of calving) displayed different behaviours compared with their healthy herdmates (normal blood calcium levels ≥2.0 mmol/L) (Figure 3).
Cows with severe hypocalcaemia:
spent longer lying on the day of calving (+2.6 hours) and the day before calving (+1.4 hours);
had more transitions between lying and standing positions on the day of calving; and,
took fewer steps on the day of calving.
The graph on the left shows the average time cows spent lying per day in the 3-weeks leading up to calving and the 3-weeks after calving for cows that had hypocalcaemia (blue) or were healthy (black). The graph on the right shows the average number of steps cows took per day before and after calving.
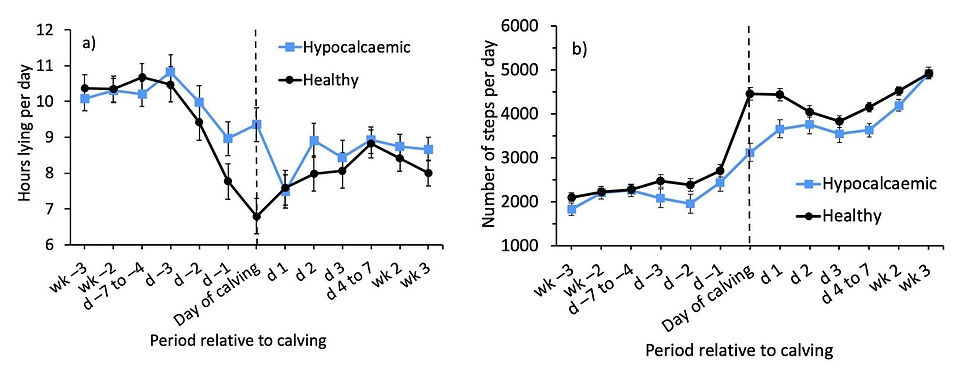
Overall, cows with severe hypocalcaemia spent more time resting and were less active immediately around the calving event, whereas cows with subclinical ketosis (in the first study) spent less time resting and were more active up to 3 weeks before calving.
Our results indicate that sick cows change their behaviour in different ways depending on type of metabolic disease. Future research is needed to build upon our work to determine if these changes in behaviour in individual cows could support the development of alerts that would signal to the manager that a cow requires additional assistance.
Are you enjoying this summary? Have a read of one of these while you're here!
Other potential uses for wearable behaviour-monitoring devices
Numerous studies have investigated whether changes in cow behaviour could predict onset of calving or detect a heat event using behaviour-monitoring devices. Devices that monitor altered behaviours in individual cows may allow farmers to receive alerts in real‑time that could signal an animal that is due to calve, is sick, or is on heat, especially if behaviour changes are not mirrored by herdmates.
Predicting calving
As can be seen below, subtle changes in the lying behaviour and activity of dairy cows occur up to 2 days before calving, with larger changes observed within 24 h of calving (Jensen, 2012). These changes in behaviour occur due to the cow ‘nesting’, becoming restless, and experiencing discomfort as calving approaches (Huzzey et al., 2005). In a recent study, we characterised the lying behaviour and activity of clinically healthy grazing dairy cows during the period immediately surrounding calving (Figure 4) using a leg-attached device (Figure 1a).
We determined from the Figure below that from 2 days before calving:
cows spent less time lying;
steps taken increased; and,
there were large increases in the number of times cows transitioned between standing and lying
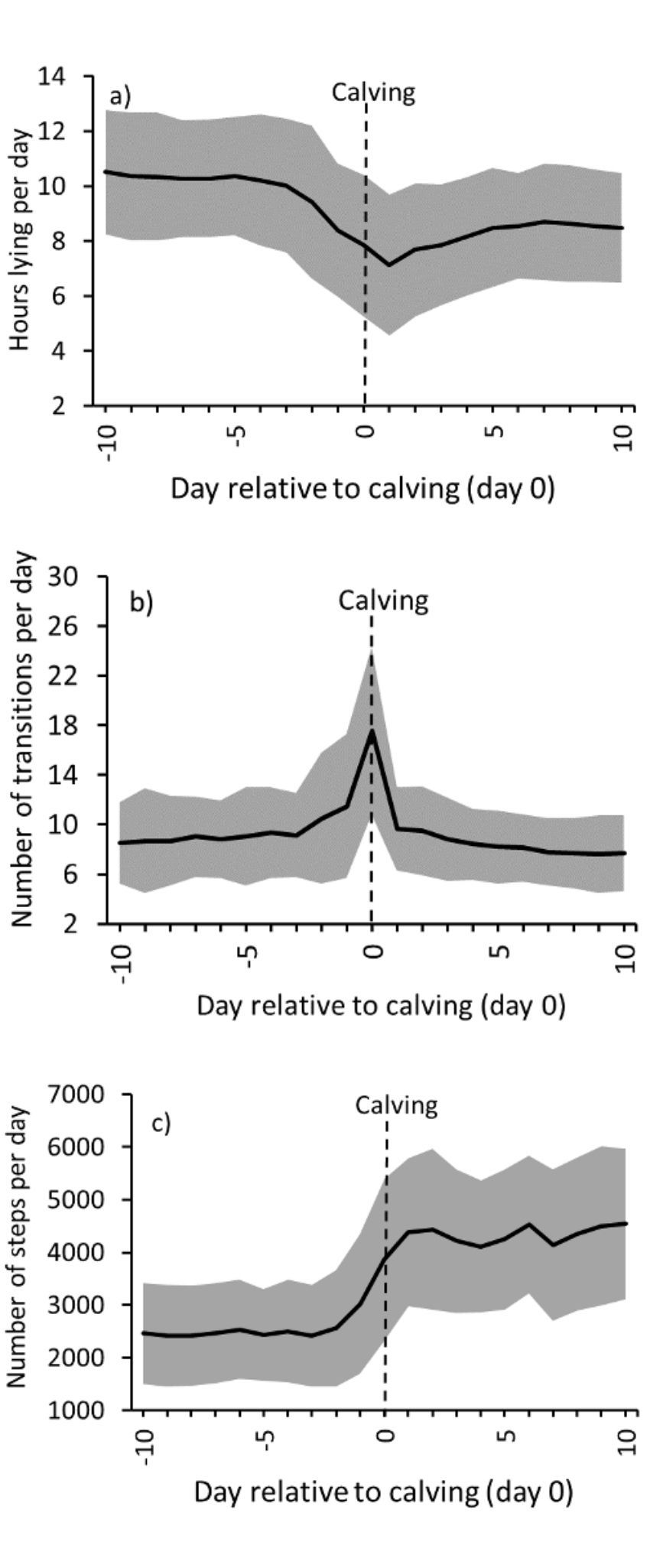
These are promising findings; however, further work is required to develop predictive tools suitable for farmers to detect calving cows in grazing systems. Overseas research in housed cows indicates that a combination of behaviours may need to be monitored to obtain the most accurate predictions of calving.
For example, researchers using leg-worn devices to record lying behaviour demonstrated that adding a second device measuring rumination time allowed calving to be predicted within 8 h (Borchers et al., 2017). Nevertheless, fitting two technologies to each animal is unlikely to be feasible on commercial dairy farms, so other more practical options need to be developed.
Heat detection
During the mating period, it is common practice to observe individual cow behaviours (e.g. bulling, standing to be ridden, restlessness) and use aids (e.g., tail paint or heat mount detectors) to assist in the detection of heat. Timely and accurate heat detection is an important skill for farmers to maximise their herd’s reproductive performance.
Recently, wearable devices that monitor activity only, or activity in combination with other characteristics (e.g., rumination time), have been studied to provide alternative, automated heat detection tools.
Automated heat detection devices monitor activity because cows on heat typically walk more as they are restless, mounting and being mounted by other cows. Some devices also monitor rumination time as cows on heat spend less time feeding. However, these devices need to be sufficiently accurate to ensure that increased activity unrelated to a heat event does not trigger an alert (e.g., cows walking to a paddock that is far away from the farm dairy).
Typically, the change in activity for an individual cow is compared with her own baseline activity and some, more accurate, systems also compare cow activity to the rest of the herd on the same day. A number of criteria must be met for the change in activity to be considered a heat event (e.g. a change in activity of sufficient magnitude and duration must occur, Figure 5). In this regard, a combination of increased activity and decreased rumination time can also help improve the accuracy of heat alerts.
Although several studies have demonstrated that wearable devices allow cows on heat to be automatically identified with a good level of accuracy and precision, some cows will still be incorrectly identified or missed (Kamphuis et al., 2012). Furthermore, the technology can fail on an individual cow’s monitor and the whole system can go down in a power outage or internet failure. As a result, relying solely on automated heat detection may not be a viable option, but it may complement other heat detection aids to assist farmers with lower heat detection performance, or if they have large herds.
Concluding messages
Using behaviour-monitoring devices during the mating period can aid heat detection and reduce labour required for this task. During the transition period, wearable devices also have potential to predict calving onset and disease, which may lead to more successful interventions, treatment and management of at-risk and sick animals. Further research is required to demonstrate that these devices can work effectively on commercial farms for these purposes, and to determine their value proposition to improve farm productivity, profitability, and animal health and welfare.
What did you think about this week's post? What other content would you like to see more of on The AgriSciencer? Leave us a comment or send us a message on our Facebook page (@agrisciencer) or in the comments below.
This work was funded by New Zealand dairy farmers through DairyNZ Inc. and by the Ministry of Business, Innovation, and Employment (MBIE). Stacey Hendriks is also supported by the Colin Holmes Dairy Scholarship and the Ministry for Primary Industries Postgraduate Scholarship. We would like to acknowledge Stacey's PhD supervisors and co-authors for her publications:
Claire Phyn, Senior Scientist, DairyNZ
Danny Donaghy, Professor of Dairy Systems, Massey University
John Roche, Professor, University of Auckland
Main References Used:
Hendriks, S. J. 2020. Behavior changes in grazing dairy cows during the transition period are associated with risk of disease. [Unpublished doctoral dissertation]. Massey University.
Hendriks, S. J., J. M. Huzzey, B. Kuhn-Sherlock, S-A. Turner, K. R. Mueller, C. V. C. Phyn, D. J. Donaghy, and J. R. Roche. 2020. Associations between lying behavior and activity and hypocalcaemia in grazing dairy cows during the transition period. Journal of Dairy Science, in press.
Comments